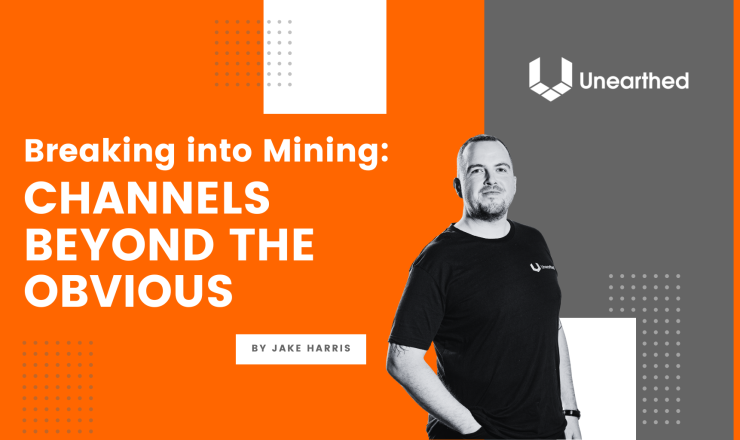
Breaking into Mining: Channels Beyond the Obvious
By Jake Harris Selling into mining isn’t always straightforward. Many innovators assume that mining companies, particularly the
Read articleOptimising Platinum Recovery through Machine Learning
Unearthed Sydney was Unearthed’s biggest event to date, with 18 teams creating exciting and innovative prototype solutions to resource sector problems. After a long and tense 54 hours, the first prize was claimed by Lachlan McCalman, Simon O'Callaghan, Alistair Reid and Daniel Steinberg of the "Statbadgers" team.
The Statbadgers came first with their prototype, the Orecaster, which forecasts platinum recovery using machine learning. We recently sat down with Lachlan McCalman to find out more about the team’s hackathon experience.
Q. Could you give us a quick description of your prototype and of the approach that you took?
A. First, we decided to attempt the challenge [Anglo American: Data Driven Optimisation of Platinum Recovery] that best played to our skills, involving difficult predictions and lots of data. We then tried to develop a basic solution quickly and iterate as much as possible over the weekend. We’re all experienced in probabilistic prediction and machine learning so we focused on applying familiar algorithms from those domains.
Q. In one sentence, could you describe the backgrounds of each group member?
A. All four of us have PhDs in Machine Learning from the Australian Centre for Field Robotics [part of the University of Sydney] and we all now work at NICTA.
For my undergraduate degree, I studied physics, Alistair did physics and engineering, Simon did electrical engineering and Dan did mechatronic engineering and commerce. We all ended up in machine learning via robotics and actually met in the lab while we were doing our PhDs.
Q. Describe your experience working in the resource space. Did you encounter any challenges?
A. We have previously done some work in the resource space through NICTA. The great thing about the mining industry is that they have a lot of data, and definitely understand the potential power of that data to improve their business.
The main challenge for us is communication. The mining companies tend not to have expertise in mathematics and statistics, while we lack expertise in mining engineering. It can be difficult to collaborate.
Q. What impact could your prototype hold for the industry? Do other industries use this current approach?
A. Our prototype implements data-driven, probabilistic modelling techniques and some industries do already use this approach. Ultimately, the biggest benefit it could have for the mining industry is enabling automatic optimisation and control of sensing, mining and refining. Critically, such control would be driven by quantified risk thresholds for the company.
Low-level operation could be handling programatically, informed by a risk-reward trade-off chosen by humans.
To summarise, the impact is all about better prediction, quantified risk and automation.
Q. What do you see as the potential future of your prototype?
A. We’re currently in early discussions with Anglo American in applying these approaches to the work that they do. Our goal is not to sell the prototype itself, but to help companies understand how our machine learning capabilities can help their business.
Q. Finally, what tips can you give to future participants at Unearthed Hackathons?
A. If you want to win, be mindful that the winner is chosen by judges who may not have the same (technical) background as you. This means that you have ensure that as well as doing the work, you’re able to communicate it concisely and explain its importance in simple terms.
Be conservative with your planning. You’ll get less done than you think. We also believe that hackathons are not good opportunities to learn to new things – there just isn’t enough time to learn something and use it! You should be going with a game plan that favours techniques and technologies with which you are intimately familiar.
Related News:
• Startup Smart – Unearthed hackathon wants resourceful problem solvers