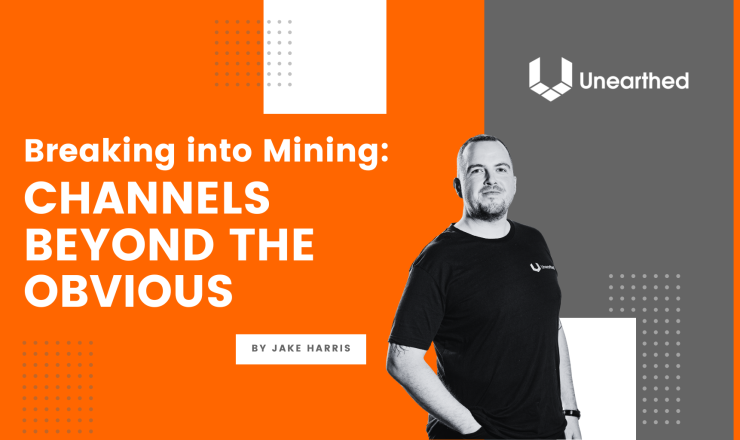
Breaking into Mining: Channels Beyond the Obvious
By Jake Harris Selling into mining isn’t always straightforward. Many innovators assume that mining companies, particularly the
Read articleExploreSA: The Gawler Challenge is a data science challenge run in partnership with the South Australian Government and Unearthed, that has attracted more than 2000 registrations from over 90 countries from around the world.
The Gawler Craton is one of the world’s most significant iron oxide, copper-gold regions and by applying creative and innovative analytical approaches to geophysical data we are potentially one step closer to uncovering the next Olympic Dam or Carrapateena.
The Data Prep Prize was created to encourage participants to share data cleaning approaches and cleaned data sets. This work helps everyone save time on their data prep, allowing participants to focus on building and testing data models.
Data cleaning is a fundamental and crucial part of applying machine learning to exploration data. Some data scientists estimate that 90% of their time is spent on data preparation, over multi-month long projects. Data cleaning is so important and time consuming, that many are building proprietary data cleaning algorithms and pipelines.
Thank you for all the submissions and congratulations to the four winners taking home $5,000 each of the overall $250,000 prize pool. Now, let’s dive in and find out a little more about the winners.
Michael Rodda is the Founder of Caldera Analytics, based in Melbourne. Michael and his team won 1st place and $500,000 in the Unearthed OZ Minerals Explorer Challenge in 2019. Prior to winning the Explorer Challenge, he worked as a data analyst at NAB. After winning, realising the opportunity for applying machine learning to exploration, Michael founded Caldera Analytics, to build a solution for the mining industry.
Michael’s submission for the Data Prep Prize is a comprehensive and easy to understand the process for removing errors from geochemistry data, prior to using machine learning applications. Modern analytical techniques such as machine learning can ingest millions of rows of data at once, automatically, which means the data errors have the potential to cause significant problems in the modelling process, which can result in the really useful data, like anomalous values of economic metals, being concealed.
One of the key differentiators of Michael’s submission was the step-by-step approach that is easy to follow for both experts and people new to machine learning. It’s an excellent guide, combined with a cleaned, usable dataset, for anyone looking to use geochemical data in their predictions.
“Data quality is a really important issue for applying machine learning to mineral exploration - a lot of the data sources may appear to be good quality on the surface but underneath there are plenty of issues that will derail a data science project. By showing how to deal with the geochemistry data entry errors, it should encourage data scientists to dig deeper into the data and make higher quality submissions.” - Michael Rodda
Russell has teamed up with Ahmad Saleem, a research analyst for a capital mining fund and the well known producer and voice of Exploration Radio, and Tyler Hall, who is completing his PhD in geoscience at Stanford University in the United States. Together, they have formed a startup and team for the challenge, Incerto Data.
For their submission, Incerto Data tackled some of the meaty issues surrounding using exploration data for machine learning. One of the biggest problems is handling different sample populations, which is also an issue for standard analytic approaches. Particularly with large geochemistry databases, like the one for ExploreSA, there are many different sample types, analytical methods and detection limits. Incerto’s approach is a user-friendly process to deal with these multiple populations quickly and efficiently. Of course, they provided the final cleaned dataset output as well.
Machine learning also requires multiple layers of geospatial data to be stacked. Incerto provides an easy way to combine point geochemical data, with geophysics data, so it can be ingested into machine learning models. For those considering applying ML to exploration, a key question is whether to use a classification or regression approach.
“As a team, our intention behind taking part in ExploreSA was to showcase ways of combining domain expertise in mineral exploration with proven data science techniques. We are currently developing products in this space and saw the opportunity to take part in this challenge as a way of honing our skills. - Russell Menezes
“As a geologist, visualising is understanding. So structuring geological data in a way that can be quickly plotted, mapped or modelled is a good way of not getting lost while undergoing cleaning and analysis on large datasets.” - Jack Maughan
Liang is a full-time Software Engineer/Geospatial Developer with a focus on geospatial software, making his skill sets particularly applicable for ExploreSA. Liang formed the TriPandas team with Ouyang is an experienced Data Scientist and Business Intelligence Developer, and Liu Wei, a Data Scientist from China.
TriPandas focused on processing multiple data types for use in machine learning approaches. Some of these datasets are quite unfamiliar to many data scientists, so the submission was a great way of allowing people to get their heads around processing fairly unique data types like Magnetotellurics (MT) and spectral remote sensing data.
TriPanda clearly documented their process and rationale for others to use, as well as all the processed datasets saving other participants significant time.
“We wish our post could save other team’s time and allow them to spend more time on the enjoyable part - modelling. We also provide an example to do our work at the free Google Colab to help those people who struggle with computing resources.” - Liang Chen
ExploreSA: The Gawler Challenge is run in partnership between the South Australian Government and Unearthed. So far more than 1700 of the Unearthed Community from around the world have signed up for the challenge.
“While businesses globally are restricted by the measures adopted to reduce transmission of the virus, the South Australian government has remained focussed on ensuring the state is in the best possible position for economic recovery once this health emergency is over. ExploreSA: The Gawler Challenge is an important facet of this rebound.” - Minister for Energy and Mining Dan van Holst Pellekaan.
The Gawler Craton is one of the world’s most significant iron oxide, copper-gold regions and by applying creative and innovative analytical approaches to geophysical data we are potentially one step closer to uncovering the next Olympic Dam or Carrapateena.
The exploration industry has been struggling with using traditional software and approaches, and I’m confident this will be a game-changer for using open datasets.
ExploreSA: The Gawler Challenge has little more than two months before the main competition closes on 31 July. The Grand Prize, Student Prize, and many other categories will be announced in September, at which point all targets and data generated by the teams will be publicly available.
Love data science, machine learning or geology? Join this epic $250K challenge to find South Australia's next big mineral deposit. ? Register Now!