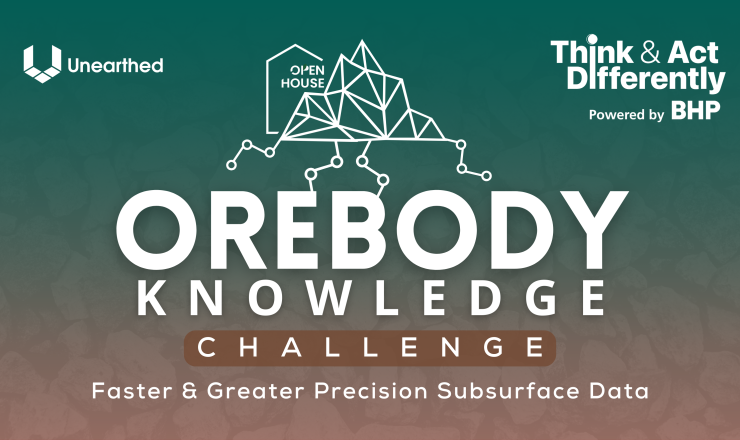
Think & Act Differently, Powered by BHP, launches global innovation challenge focused on Orebody Knowledge
Unearthed, a leading facilitator of open innovation in the resources sector, is pleased to announce the launch of the latest
Read articleThe Gawler Region of South Australia is one of the world's most prospective geological domains. It holds deposits such as BHP's Olympic Dam and OZ Minerals Prominent Hill and Carrapateena copper-gold deposits. Data scientists and geoscientists from over 100 countries have competed for months to generate new solutions for mineral exploration.
The ExploreSA: Gawler Challenge reflects an appetite for data science to take a more prominent stand alongside traditional mineral exploration techniques, where emerging methodologies can mitigate risk, optimise outcomes, and deliver targeted exploration strategies. he outcomes of the challenge signal to investors that South Australia is ready and willing to facilitate large-scale mining operations and the economy will benefit from the activities surrounding the multi-million-dollar projects that may arise from the results of this challenge.
The novel solutions presented by challenge participants have proven to be diverse, with many targets generated using multiple methods. These results are captured in an interactive map, with the full results of the challenge now available from SARIG.
Read also: Data Prep Prize Winners for ExploreSA: The Gawler Challenge Announced
Team Per-Oz is a collaborative effort by Paul Pearson from Latin Global and John McLellan from GMEX. They specialise in structural geology, prospectivity analysis, data science, machine learning and modelling for the global mining and exploration industry. Paul is based in Lima, Peru, and John is based in Queensland, Australia. Both work as industry consultants.
A data-driven methodology (machine learning) that systematically tests for the relative importance of a large number of geological factors on known deposits. The approach integrates a mineral systems approach that emphasises and analyses the processes operative in the passage of ore fluids and metals to their eventual deposition sites. Per-Oz identified essential ingredients in the different crustal levels of the theoretical mineral system as 100 mappable criteria in the exploration data. These criteria are input into the machine learning algorithm and were used to make predictions about the less known areas. Additionally, an analogue-driven methodology to test geological processes (geomechanical modelling) was applied that simulates rock failure and ore fluid flow at potential metal trap sites in the upper crust to hone district-scale targeting further.
So how do Drs Paul Pearson and John McLellan see their solution working in a production environment?
We think that the information sets we have provided encompass both the Data Science and Machine Learning approaches but have remained strongly focused on the Geology, which we think is integral to providing a meaningful information base that has highlighted the best prospective targets in the region. Our methodology is clear, in that we focus on the critical geological factors when supervising the machine learning and combining this with one of the most important 'ore-localiser' factors, that being the deformation history and the geomechanical response of the upper crust. This approach will benefit the explorers by encouraging them to think about how the deformation events have provided key locations for fluid focusing and mineral deposit formation.
The Judging Panel was impressedby Per-Oz's novel approach that showed effective integration of mineral systems and geomechanics, with appropriate machine learning techniques, that was presented with superb technical quality. The judges noted that the submission from Dr John McLellan and Dr Paul Pearson would be very accessible and useful to explorers of the Gawler region.
Caldera Analytics is a data science consultancy formed from the winners of last year's Explorer Challenge. Caldera is focused on using machine learning to aid explorers in looking for base metals undercover, lowering the risk of exploring in these challenging areas.
Caldera Analytics created a machine learning model that predicted prospectivity of basement hosted IOCG deposits in the Gawler Craton. Every input dataset had to be fit for purpose; every feature had to be backed by a solid rationale, and no predictors with tenuous correlations were included. After extensive investigations, the only fit for purpose datasets useful for broad-scale prospectivity mapping are gravity, magnetics, magnetotellurics and crustal-scale faults.
Modelling was done in a conservative spatial validation scenario, ensuring that it could operate reliably no matter how unique the geological terrain was. Broad-scale prospectivity regions from the model indicate that the Mabel Creek region and the Peake and Denison region should be taken more seriously for IOCG mineralisation, supporting the recent developments from Petratherm and Minotaur in respect to these areas. Mabel Creek is the most interesting area due to the presence of a magnetotelluric conductor that goes further north into unpegged, low gravity survey regions, providing new opportunities for all explorers.
So what advice does Caldera Analytics have for emerging innovators?
Focus on being transparent as possible and don't ignore simple machine learning models. Creating effective models that generate business value for explorers will always be better than making a brilliant but hard to follow analytical approach.
The Judging Panel noted that Caldera Analytics' submission featured a robust discussion on what data was used and why - including scepticism around ML approaches. They were also commended for using negative drill holes as training data and an innovative application of gravity data, which is practical for data scientists in exploration to use.
Sparveon represented an international student team that developed machine learning and big data solutions to track the patterns of satellite imagery and numerous geological datasets, which they hoped could rapidly and precisely generate the prospective target area of mineral and energy resources.
Submission Summary
A data-driven methodology (machine learning) that systematically tests for the relative importance of a large number of geological factors on known deposits. The approach integrates a mineral systems approach that emphasises and analyses the processes operative in the passage of ore fluids and metals to their eventual deposition sites. Sparveon identified essential ingredients in the different crustal levels of the theoretical mineral system as 100 mappable criteria in the exploration data. These criteria are input into the machine learning algorithm and were used to make predictions about the less known areas. Additionally, an analogue-driven methodology to test geological processes (geomechanical modelling) was applied that simulates rock failure and ore fluid flow at potential metal trap sites in the upper crust to hone district-scale targeting further.
So, what did team Sparveon think of the ExploreSA challenge?
If we could define ExploreSA: Gawler Challenge in one sentence, it would be 'Challenging yet fun'. Having the opportunity to participate in this challenge is the greatest thing that could happen this year. Gawler Challenge not only provides the opportunity for us to experience data, but also the community where you can collaborate your ideas with other participants, discuss your problem, and might as well get the answer. The community this competition builds motivates us to challenge ourselves even further.
The Judging Panel was impressed by the strong discussion on what data was used and why, including scepticism around machine learning approaches, alongside the use of negative drill holes as training data and the clever application of gravity data.
The DeMIST team gathers expertise in geoscience and machine learning from all corners of the Earth. DeMIST works together in the historic mining town of Freiberg and have a shared passion for applying cutting edge Earth Science to ensure sustainable use of mineral resources.
Submission Summary
DeMiST characterises the potential generation and transport of metalliferous fluids at a regional scale, based on the iron-oxide-copper-gold (IOCG) mineral system, and then targets trap structures in fertile areas that may have accumulated economically significant amounts of copper and/or gold.
DeMiST applies multiple supervised and semi-supervised machine learning approaches and integrates their predictions using a Bayesian framework to identify six high-potential regions in the Gawler Craton. Prospective trap structures were then mapped to identify surface and subsurface anomalies consistent with the distal footprint of a mineral deposit. This workflow enabled them to identify high potential targets with
a fertile crustal setting conducive to the generation and transport of mineralised fluids,
appropriate structural traps to trigger metal precipitation and the formation of a deposit, and
anomalous surface or subsurface geochemical data typical of the distal footprint of IOCG deposits.
Does DeMIST have any advice for emerging innovators for creating successful submissions?
There is no substitute for understanding the datasets you're working with and how they were collected. The devil is in the detail, and even the fanciest machine learning algorithms can be easily biased by errors, unbalanced data and noise. The Earth is shaped by complex interactions between natural processes that are variable in time and space, so an understanding of mineral deposit formation is needed to DeMISTify available data and successfully apply ML to minerals exploration.
The Judging Panel commended DeMIST for their multi-scale approach, a good combination of geological and machine learning approaches, and a multi-step machine learning approach that incorporated feedback.
Jack Maughan is a geologist by training and a self-taught data scientist currently based in Adelaide, South Australia. Jack's experience as a geologist includes exploration and production roles in Cu, Au and U, in both Australia and Canada. More recently, he has developed a strong interest in data science and gained experience in "unpackaging and demystifying the black box of machine learning" with regards to mineral exploration.
Submission Summary
The Calculating Copper submission is an interactive and reproducible workflow for creating mineral prediction maps using machine learning. In particular, Calculating Copper uses regional drilling results, geophysical and geological data over the entire Gawler Craton to predict areas of base metal mineralisation (Cu, Zn, Pb, Au) using a mineral systems approach. Multiple mineral prediction maps are created utilising and comparing various machine learning algorithms. Models are validated using existing mines/deposits and then used to propose future drillhole locations.
So how does Jack envision explorers benefitting from his model?
I hope that explorers can benefit from my submission by breaking down my methodology and building upon it, customising the workflow to suit their own region or target specific needs. Providing explorers with an understanding and thought-provoking insight into machine learning is the main aim of my submission, so I hope that it can be a basis from which more ideas can grow.
The Judging Panel praised Jack's combined approach that considered variables that other teams didn't, such as depth to basement. It was noted that Jack's approach would be a beneficial foundation for those looking to apply machine learning to targeting activities.
Sam Bost submitted an individual submission for the GawlerSA Challenge. Sam currently works in production operations and on advanced analytics projects as a Production Engineer at Shell, as well as on advanced analytics projects as a Machine Learning Engineer at SIG Machine Learning Pty Ltd. For the GawlerSA challenge, Sam expressed his gratitude for the support and collaboration from the Unearthed community, as well as friends and colleagues across the globe involved in geoscience and mineral exploration. Working at SIG, Sam is passionate about empowering individuals and businesses by identifying new opportunities to gather, transform and model their data to deliver safer, smarter, simpler ways of working. Sam also has a great sense of humour, and a very cute dog.
Submission Summary
A novel approach to predict the location of mineral deposits in the Gawler Craton region using data-driven, deterministic deep-learning models. By adopting an integrated and collaborative approach, this project considers several data sources, including geophysical surveys, remote sensing satellite imagery, geochemistry analysis, geological mapping and subsurface drillhole data. In particular, the Gawler Craton Aerial Survey (GCAS) was found to be a key data source that delivered exceptional results when implemented in machine learning (ML) models. This project delivers three regression-based supervised machine learning models. These models are packaged into a single artificial intelligence (AI) software, aptly named 'Minny'.
What has impressed Sam the most about the ExploreSA: Gawler Challenge?
With the GawlerSA challenge as my first involvement in an Unearthed Competition, I was blown away by the willingness of the ExploreSA community to collaborate and share learnings. As a petroleum engineer, I found the introductory videos and the data prep submissions incredibly helpful to find my feet in understanding how to utilise the available data set to deliver insights to take forward in mineral exploration.
Aside from a great presentation video, the Judging Panel commended Sam's novel approach that featured a good combination of machine learning and geology, generating independently verified targets.
Avant Data Solutions is a small machine learning consultancy with experience working in the mining/exploration and process spaces. Avant's focus is on image processing, mineral target prediction, and process optimisation.
Submission Summary
A neural network model was produced to output prospectivity maps for targeted commodities in the Gawler Craton. This model was trained in several stages, transferring learnings from one stage to the next.
The first stage was self-supervised training, without requiring any examples, using datasets to their fullest extent to maximise data utilisation. In the second stage, the model is updated with Australia-wide occurrences as targets, producing a large-scale mineral prospectivity map. The intent is to distil patterns from a larger domain of geological contexts into the model. Lastly, the model is targeted to specific mineralisation types within the Gawler Craton.
Geochemistry is used to create an alternative "assay-guided" output with a significantly increased targeting ability; however, it is only valid in areas in the vicinity of existing assay results.
How does Avant Data Solutions think explorers can use or benefit from their submission and methodology?
We think the value of our solution is the ability for explorers to get a head start in looking at areas of higher value at the front end of exploration. This allows regions to be screened quickly and at scale, allowing more time for the exploration team to de-risk and identify targets for further exploratory work.
Avant Data Solutions was commended by the Judging Panel for their model-training using an Australia-wide dataset, which effectively generated greenfields targets. This team was also noted for having considered the economics of their approach, and for including the weightings around their predictions.
If you have any questions about the ExploreSA: Gawler Challenge, or would like to create a challenge of your own, please contact us by emailing [email protected].